
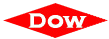




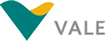

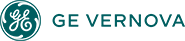




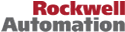

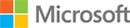
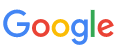

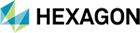


Although operations and maintenance activities typically generate vast quantities of data – both structured and unstructured – operations and maintenance groups can usually only leverage a small percentage of these data to make better decisions. Most operational data from systems are stored within a process historian archive, or not at all. Prescriptive maintenance can help users who need the data to predict and respond to operating conditions or poorly performing assets.
To be able to more accurately predict the performance of their industrial processes or assets, companies are beginning to invest in new process analytics solutions that combine either time-series or streaming sensor data with machine learning algorithms.
To improve asset performance and availability, process analytics must learn the machine or process, identify specific data patterns and search time-series data over a specific timeline and correlate all related events quickly and efficiently. This includes the time-series data generated by control systems, maintenance, systems, and lab systems, along with observations made by operators and engineers.
At the 2016 ARC Industry Forum in Orlando, Yousef-Abdul Moty, Director of Locomotive Engineering with CSX, presented the company’s long-term strategy for predictive maintenance. CSX, a US-based rail transportation company, operates 21,000 miles of track (fixed assets) and a fleet of 4,000 locomotives (mobile assets).
In 2004, CSX changed its business strategy to focus on profit drivers and the competitive advantage the company could achieve by leveraging newly available technology.
With the knowledge that the value of the company’s operating assets – mainly comprised of locomotives, cars, and track – exceeded $60 billion, corporate eyes quickly turned to asset utilization. With replacement costs in the neighborhood of $1 million each, locomotives represented the largest cost pool to address. In addition, the company’s rail infrastructure – with some rails dating back to 1902 – was starting to show its age.
By tracking company financial results between 2013 and 2014, the company was able to identify strong evidence of an increase in revenue and profit, which Mr. Moty attributed to asset utilization and technology enablement.
Prior to this, CSX had embarked on internal and external benchmarking activities that began when Mr. Moty attended a Berlin InnoTrans Conference where he came to the realization that Europe was much further ahead of North America in terms of enterprise asset management (EAM). CSX teamed up with ARGO, a consulting firm, to look at internal processes and uncovered surprising results.
This study revealed that CSX’s expensive locomotives typically only spent 40 percent of the time actually pulling freight; the only time the company makes money. In addition, the company learned that its employees were only productive 23 percent of the time, a staggering statistic. This internal analysis revealed over $100 million in potential savings; driving CSX to shift its focus to improving asset utilization to improve operating ratio and return on assets. This involved several parallel initiatives.
According to Mr. Moty, the most important way to get organizations to rally behind a shift in maintenance, or change culture, is to tie these programs to compensation. CSX successfully tied a long-term incentive program for executives to operating ratio and return on assets. This new focus on return on assets (ROA) drove CSX to develop new measures for asset performance. The company’s Asset Performance Index (API) describes how much of the time a particular asset is making money and how much time it is unproductively sitting around at a maintenance facility or terminal. The company also created a train delay index to better understand the severity of a trip failure and the cost attributable to delayed trains.
CSX’s core business is to safely and reliably transport customer goods on time. However, the company was previously plagued by operational and catastrophic failures. CSX reliability professionals knew the performance-to-failure curve all too well and since 2003 the company had experienced over $1 billion in total losses in 2,500 train derailments due to human factors, tracks, locomotives, and other causes.
CSX started its journey by initiating a series of projects designed to enable the company to effectively predict and more rapidly resolve (or avoid) issues with its extensive fixed assets (tracks) and costly rolling assets (locomotives and rail cars.) But first, it needed to identify an appropriate partner. In 2014, following a careful review of several suppliers in this space, CSX selected Mtell, a leading supplier of machine learning technologies for industrial assets.
To be able to identify and remediate track-related issues that could cause derailments and wheel or bearing damage on the company’s fleet of locomotives and freight cars, CSX deployed a series of interconnected sensors and wayside systems that continuously study the car movements throughout the US East Coast. CSX calls these “super-sites.” As a locomotive and the entire train pass through these stationary monitoring sites, the system reads the acoustic signatures of the bearings, peak impacts/pounding on the rail (indicating out-of-round wheels), and looks at lateral forces (geometry) such as wild or hunting trucks. Automatic equipment identification (AEI) sensors count the axles to let rail maintenance crews know exactly where repairs are needed.
This IIoT-enabled monitoring and predictive analytics system, developed in conjunction with Mtell, helped CSX drastically reduce the incidence of derailments and wheel- and bearing-related failures. The key was to be able to identify the point of degradation, “Point A,” on the PF curve and prevent both “Operational Failure B” and “Catastrophic Failure C.” In other words, catch the failure before it happens and increase the notice time.
Two percent of the company’s 80, $100,000-plus locomotives were failing each year. CSX decided to embark on a three-phase pilot project with Mtell to determine whether oil analysis could be used to predict costly locomotive failure in time to prevent these failures.
Previously, the company had been performing simple threshold analysis on engine oil samples to alert personnel of issues and initiate workflows with some moderate success. But it suspected it could do more.
To begin the pilot program, CSX provided Mtell with existing oil sample data to analyze for potential failures or anomalies. The samples for these data were random in nature and each included a variety of wear metals, viscosity, and total base number.
CSX did not provide Mtell with relevant details, explanations, or backup materials about these samples from 472 locomotives. Mtell chose nine of these samples for closer examination. These nine samples contained a total of 22 data tags from oil and maintenance records. This analysis identified eight distinguishable patterns as failures. Shown below is the Mtell probability wave that provided a visualization of all 22 data elements shown together in one graph for easy interpretation.
The red line showed an anomaly in the data. The event shown at the line created what CSX called a “virtual work order” to flag an event and look before and after the event.
The Mtell software revealed increases in iron, viscosity soot level, and antiwear and how the percentage of each impacted the overall signature. The Mtell software further identified 18 low-water-pressure problems on a particular locomotive, unaware in advance that this locomotive was a bad actor. While the CSX maintenance staff was fully aware of several cases of cooling water pressure failure causing crankcase bearing failure, this had not been disclosed to Mtell. This process was able to find and “train” the Mtell software agents against the failures and correlate this against maintenance history.
Once convinced by the analysis of the initial data samples, CSX proceeded to look at a fleet of 235 locomotives, 8,635 samples, and 1,754 repair records. CSX then looked at 120 known failures (crank case overpressure, engine running hot, etc.), built a model of these data with the known failures, marked the point and time and looked back in time, then provided this information to Mtell. Using these data, Mtell was able to predict failures 60 days before the failure occurred. A similar model was then built on the active locomotive running fleet. CSX was able to identify a pending failure by looking at the Mtell data. The flagged locomotive was routed to a maintenance facility for a pressure test on the cooling system. Normal maintenance pressure testing had missed the problem.
Once the pilot was extended to 600 locomotives and a new Prescriptive Performance Improvement Request (PIR) workflow initiated, CSX was able to successfully move from a “run to failure” operating model to a proactive preventive/predictive maintenance operating model; something that corporate finance had previously decided was not viable.
Several proactive maintenance failures have been identified and PIR’s created using the Mtell software, oil samples, and other data. These include high crankcase wear and contamination (grit and sand) in the crankcase using oil. Once the pilot turned CSX into believers, the company is now looking at taking the solution further through multivariate analysis using engine parameters like temperature, pressure, and vibration, in addition to the original oil sample analysis.
For the near term, CSX will continue to host Mtell internally, replicating agents and models for its entire fleet and integrating into the company’s IBM Maximo enterprise asset management (EAM) system to automatically create PIR’s within a Data Lake on SAP HANA and Hadoop. In the future, the company will integrate all locomotive communication systems into Mtell software running at corporate. This will involve over 300 sensors for each locomotive and 1,200,000 potential data points for the enterprise.
Mechanical equipment specialists can install and configure the system and manage agent preparation and deployment. This includes tasks to automate data auditing/cleansing, training agents with machine learning algorithms, and turning them into live monitoring implements that require little supervision.
As we learned in Mr. Moty’s presentation, process analytics solutions can leverage an industrial organization’s current data on top of a process historian infrastructure to detect patterns and predict both process and mechanical events and anomalies.
Machine learning is not new; its roots can be traced back to before the first computer. What has changed is the use of automated machine learning, the scale of computing power, intensely powerful algorithms that process massive amounts of data, and intuitive uses for time-series sensor data from machines and manufacturing processes. Machine learning enables a new paradigm. Now, rather than having a problem and formulating an algorithm to solve that problem, we can give the machine the problem and let the computer solve it.
Currently, two methods of machine learning -- supervised and unsupervised -- are commonly applied to data. Supervised learning is when you know the target of what you want to learn. Unsupervised learning is when you do not know the target, but want to find the normal structure of the data and make sense of excursions.
According to the company, Mtell’s use of real-time, live monitoring agents to perform this automated analysis is an industry first. The solution can provide prescriptive, predictive analytics and automatic notification, without significant interrogation from engineers or operators.
Based on these learnings, ARC recommends the following actions for owner-operators and other technology users:
If you would like to buy this report or obtain information about how to become a client, please Contact Us
Keywords: CSX, Process Analytics, Predictive Analytics, Prescriptive Analytics, Mtell, ARC Advisory Group.