
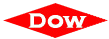




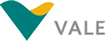

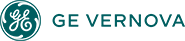




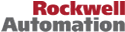

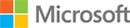
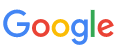

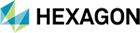


Abbott’s nutrition business, a division of the global healthcare company, manufactures a wide variety of science-based nutrition products. These range from Similac brand infant formula to adult nutrition brands such as Ensure. Recently, ARC had the opportunity to speak with James Li, an engineering manager in the Abbott Nutrition division. We discussed how the company uses Big Data and analytics to improve manufacturing productivity.
In 1999, Abbott Nutrition began working with OSIsoft’s PI System to integrate, collect, and contextualize data at the company’s manufacturing plant in Columbus, Ohio. Based on its success at this plant, in 2012 the company entered into an OSIsoft Enterprise Agreement (EA) to include all Abbott Nutrition manufacturing sites globally. This created a huge volume of data, but also presented the challenge of extracting maximum value from the data.
About two years ago, Abbott began focusing on ways to obtain more value from the data. The company used OSIsoft’s Asset Framework (AF) to contextualize and organize the data using AF’s asset-centric models. The company also started using Seeq’s advanced analytics technologies to reduce the time and effort needed to connect to AF, create models, and find insights quicker.
Seeq’s advanced analytics technology can be used to contextualize time series data and create models to help engineers quickly derive insights and value from industrial process data. Abbott decided to use the technology to help reduce clean-in-place rinse time while maintaining or improving product quality. In 2016, it started a modest pilot project on one PI Server workstation to prove ROI. According to Mr. Li, “By the end of the pilot, we felt confident in the value we could obtain and increased the project scope to include additional PI Servers.” Abbott launched a formal analytics program globally at the beginning of 2017, with near-term plans to connect Seeq to all OSIsoft PI Servers globally.
Seeq’s three-stage model includes: 1. data wrangling; 2. engineering investigation; and 3. sharing insights.
The first step, data wrangling, replaces the hours of manual effort typically required to aggregate, cleanse, and contextualize data. Data wrangling turns specific asset data into useful information “capsules.”
Capsules, a Seeq innovation, consists of data correlated by a user-defined slice of time that can be visually correlated with a unique identifier and then compared to other assets. Once defined, capsules may be overlaid on top of each other or compared side by side for analysis and visual comparison.
The second phase, engineering investigation, pertains to developing the model. Seeq’s analytics tool helped Abbott target the problem by searching and annotating, pattern matching, and historical benchmarking. This enabled the company to quickly identify and target previously unidentified problems.
The third phase, sharing insights, includes performance monitoring and reporting, dashboards, and real-time collaboration. According to Mr. Li, “Our engineers love their own spreadsheets with their own formatting. However, to improve collaboration and transfer process knowledge to newer workers, the information needs to be shared.” The Seeq tool supports the sharing of displays, called Seeq Worksheets, with a common URL; the software also exports information to Excel and their business tools for sharing and role-based dashboards. Both approaches improve collaboration globally.
Abbott Nutrition has about 14 manufacturing sites (with over 70 manufacturing sites globally for all of Abbott). The company’s goals include:
To meet these goals, the company used Seeq analytics technology in two use case pilots. The first involved CIP processes; the second focused on reducing overfills in packaging operations.
To reduce clean-in-place cycle times while maintaining or improving product quality, the company focused on asset utilization. Mr. Li explained that he wanted to be able to use Seeq to review the data stored in the PI Server to quickly identify periods of interest.
CIP is commonly used to clean process equipment in between batches or production runs. While a process is in the CIP phase, the equipment is not available to produce product. Typically, CIP is a centralized system that can connect to and be used to clean multiple process units. CIP systems typically comprise multiple circuits or steps that involve circulating caustic, acid, and water to clean the process equipment. To determine the endpoint for each CIP step, Abbott uses conductivity sensors to measure the water/acid/caustic parameters.
While Mr. Li had previously created trend reports to try to identify and isolate specific CIP issues, trend data alone does not always tell the complete story. For this pilot, he created a model using the Seeq technology to validate and improve the way the available data was used. “To be able to capture the most important data and assimilate the intelligence, we used the capsules to break down the data into phases specific to the CIP process and equipment. This information allowed us to quickly create a phase capsule with information that compares circuits and equipment,” Mr. Li explained.
The tool allowed Mr. Li to quickly identify patterns, see the duration time for each circuit and phase, identify the longest CIP run, and drill down to determine the reason for the longer run. He was also able to analyze the incremental costs accrued. The capsules helped identify excessively long CIP runs, over-rinsing, and/or when and in which CIP circuits the acid or caustic was overused. Then, he could drill down to quickly identify the root causes, such as valve leakage or other instrument failure.
The ability to quickly identify the root cause enabled the company to fix the issues to improve operational efficiencies through more consistent CIP duration. In some cases, Abbott was also able to decrease CIP cycles to increase production availability.
Another benefit was the ability to reduce product contamination by quickly identifying conductivity peaks. For example, if it was revealed that a valve leak caused a conductivity spike, an operator could put the batch on hold to prevent product contamination.
For the CIP cycle use case, the tool helped Abbott identify key areas to focus on for production improvements.
The second Seeq pilot use case focused on Abbott powder filling equipment. Here, it’s important to reduce product filling errors to enable the company to meet its filling label claims for product weight, while minimizing costly product giveaway. In the past, it was time consuming to examine the filling data and obtain batch information.
Using the Seeq toolset, Mr. Li could contextualize continuous data streams using a model that calculates product giveaway and aggregates the information over the time periods of interest. He did this by creating capsules that enabled the company to review the weight data vs. the target and filling state and compare filling operations, analyzing loss-per-fill and aggregating by product and shift. This enabled the company to determine if the target was met in about five minutes, providing a resolution to the overfilling problem.
To obtain the complete story, Abbott totalizes giveaway across shifts to find variability. It does so using dashboards in PI Coresight to visualize the data. Also, by monitoring the filler head actual weight distribution, the company can determine when filler maintenance is required.
When implementing new tools and approaches to capture knowledge, cultural considerations are important. Abbott has knowledgeable people with extensive process expertise. These experts have a lot of knowledge, but it often resides in the respective heads, laptops, and spreadsheets of its experts, as well as in PI ProcessBook trends.
Seeq and OSIsoft technologies enable the company to move to a more collaborative environment with consistent URLs. Abbott has innovation groups working globally to capture knowledge. The Seeq capsules are helping speed this process to support collaboration, innovation, and transfer knowledge and expertise to the new worker generation.
Mr. Li emphasized that the requirements will be different for different roles and it is clearly important to identify the target user and his needs with different dashboards and views. He cautioned that there is a lot of information in the data, however, analytics without context is inaccurate. “Use real-time data in context to make fact based decisions and deliver business improvement,” he recommended.
If you would like to buy this report or obtain information about how to become a client, please Contact Us
Keywords: Analytics, Manufacturing Productivity, Clean-in-place, Product Filling, ARC Advisory Group.