
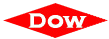




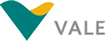

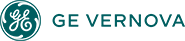




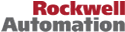

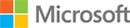
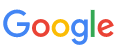

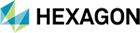


The COVID-19 outbreak created uncertainties and changes in every aspect of daily life and work. It’s also pushing business leaders and plant owners to act more quickly than ever before, to survive and outperform others. This provided more possibilities for AI technologies and has speeded AI deployment in manufacturing.
From a practical perspective, we will see more AI-based applications specifically targeted toward various aspects of decision making, such as improving production/operation efficiency, reducing downtime, providing predictive maintenance, optimizing supply chain, and reducing energy consumption.
Human and AI capabilities complement each other. Humans are creative, can see above the current task, and can apply knowledge from other experiences in a current task. However, humans are less perfect in repetitive tasks. AI is high-performance and diligent, but less creative. There is great potential for human and AI collaboration, and humans must interact collaboratively with AI as they continue to fill the roles of project owners, system trainers, final users and will interact with AI throughout the lifecycle of a project.
AI is being applied to a wide range of applications and industries. In process control, AI and machine learning are being applied to advanced process control (APC) applications and autonomous plant operations. In discrete manufacturing, AI is being applied to robotics. All processes could theoretically be controlled by some form of artificial intelligence in the long-term. Whatever the application, there are some basic steps identified by ARC to ensure success in your AI deployment:
Based on presentations from ARC’s 2022 European Forum, this strategy report provides the latest AI use cases and comprehensive comparison of strengths and challenges of humans vs. AI. This report presents how humans and AI can collaborate effectively and which key dimensions and applications to consider. Companies that supported the event include Microsoft Project Bonsai, Dow Chemical, NNaisense, ABB, and Throughput AI.
The following industrial use cases for AI technologies were all shared at the 2022 ARC European Forum.
Microsoft Project Bonsai shared their views on autonomous systems, and how the Bonsai platform can optimize equipment and processes by sensing and responding in real time. According to Microsoft, autonomous transformation is an evolutionary process consisting of four stages:
From the second stage, enterprises may use supervised and unsupervised learning to realize things like better predictive maintenance and demand prediction. The Bonsai platform combines simulation, deeper enforcement learning, and machine learning. Bonsai can help users build AI models with their own experience and industry know-how and speed up the development of the latter three stages. Below are some Bonsai case studies:
Dow Chemical shared how cloud enabled predictive maintenance via optimizing manufacturing data and analytics. Unplanned downtime accounts for vast amounts of lost revenue for the process industries. Leveraging predictive maintenance to act before failure happens is one of the leading applications for AI in manufacturing.
In Dow Chemical’s own project, three distinct persons work together as a team. Data scientists train the models and check the Azure Cloud environment. Azure Cloud developers ship plant data to cloud and feed data into models. On-site operators perform monitoring, take actions when receiving information from systems and provide feedback into the systems. This working cycle moves on continuously to build algorithms and models more efficiently. In this project, cloud computing is necessary to address a few headaches, including:
Throughput AI shared how AI helps optimize supply chain and convert visibility to action ability. Supply chain is opaque, piecemeal, and inefficient all the time. COVID-19 outbreak made its bottlenecks more obvious than ever in recent years. Meanwhile, enterprises face increasing pressures to improve supply chain efficiency and find opportunities for better business, operational, financial, sustainability results and outperform industries in the long term. As an enabler, AI can help to leverage existing data and domain expertise of the existing teams, to increase output, inventory turns, and profitability, minimize excess inventory, and reduce waste and CO2. Some cases shared here:
ABB shared details about its AI Assisted Operator to illustrate how AI could help operators. Production processes produce many alarms, and it’s easy for operators to fall victim to alarm floods or to ignore alarms that may be critical. AI is one of the latest technologies that can provide operators with more direct, efficient, and cost-effective support for effective alarm management in the long term. The AI Assisted Operator is a mix of different AI applications, its workflow includes:
NNaisense introduced details of its AI-based adaptive control system called Adaptive, Rational Core. The control system will build a modular, task-agnostic, and verifiable model. Different from traditional reinforcement learning, the system can be imposed with new goals and constraints at any time and perform tasks on command. The system cannot only help control the plant, but also predict the future in various scenarios and plan backwards in time from target state to the current state.
Human and AI collaboration happens all the time. All AI-based systems need to go through many iterations of validation and verification alongside continuous interaction with plants and the outside, or when taking on new tasks or missions. Humans will go through five stages interacting with AI-based systems:
Most people may be curious about how AI technology is progressing in practice. Based on the poll in the ARC Forum, “first products and solutions available,” “R&D” and “beta testing/selected projects” are the common stages among participants. Generally, it’s still in a nascent stage.
As our expert panel discussion revealed, companies at the beginning of their AI journey struggle to define a strategy for successful implementation of the technology. Many companies in the early technology evaluation stage go for a trial-and-error approach. However, a strategy is critical to formulate the KPIs and can be necessary to get management funding and continue the AI journey. As these journeys can last quite long it is important to have the strategy, goals, and KPIs right, as management teams can change, and this can bear a threat of discontinuation.
ARC Advisory Group clients can view the complete report at ARC Client Portal
If you would like to buy this report or obtain information about how to become a client, please Contact Us