
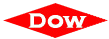




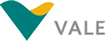

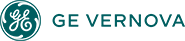




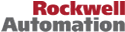

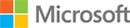
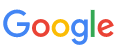

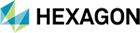


In the ever-evolving landscape of Artificial Intelligence (AI), generative AI has emerged as a key player, promising to revolutionize industries and business processes. However, it's not without its share of complexities and confusion. To shed light on this innovative technology, I committed to explore the latest breakthroughs and their applicability to Industrial AI with a set of ARC blogs, podcasts, Insights and Reports. However with the general confusion being created by generative AI myths and misconceptions, I've worked with the team at ARC to lay some AI foundations, including a glossary of terms, in our initial report on The Industrial AI (R)Evolution.
Industrial AI, a subset of the broader field of artificial intelligence (AI), refers to the application of AI technologies (including Generative AI) in industrial settings to augment the workforce in pursuit of growth, profitability, more sustainable products and production processes, enhanced customer service, and business outcomes. Industrial AI leverages machine learning, deep learning, neural networks, and other approaches. Some of these techniques have been used for decades to build AI systems using data from various sources within an industrial environment, such as sensors, machinery, industrial engineers, and frontline workers.
Among these AI techniques and technologies, generative AI has caught the attention of many, particularly within various industries such as Aerospace & Defense, Automotive, Electric Power & Smart Grid, Industrial Equipment, Oil & Gas, Semi-Conductors, and more.
Generative AI, powered by machine learning and neural networks used for decades in various Industrial AI use cases, but with new genuine breakthroughs in natural language processing (using GANs, transformers and LLMs), are revolutionizing how we interact with everything around us, whether those technologies are inherently smart, industrialized, or not.
However, this revolutionary technology often leads to confusion due to general media hype about AI, extravagant marketing claims from software suppliers struggling to get due credit for having invested in AI technologies long before the current wave of generative AI breakthroughs, its technical complexity and the rapid pace at which AI continues to evolve.
The current state of industrial AI presents a complex picture. On one hand, we have a myriad of AI solutions promising to revolutionize processes and boost efficiency. On the other hand, there is a lack of clarity regarding which technologies truly deliver on these promises.
One of the main challenges faced by organizations is discerning valuable AI breakthroughs from the hype. While many AI technologies have proven their worth, others are still emerging, and their long-term value remains uncertain.
Moreover, there are numerous myths and misconceptions surrounding which of these AI techniques and technologies relevant to industrial AI use cases. These include the belief that AI implementation requires massive upfront investment or that it will lead to widespread job displacement. Such misconceptions often deter organizations from exploring the potential benefits of AI.
To address this confusion, the ARC Advisory Group has embarked on a mission to simplify the complex, identify relevant breakthroughs, and cut through the hype surrounding industrial AI. Central to this mission is The Industrial AI (R)Evolution that cuts through the Generative AI hype, dispels myths and summarizes the latest developments and trends in the field.
The report covers a wide range of topics, including data governance, cybersecurity risks, high-value industrial AI use cases, and the societal impact of AI. Additionally, it dives into the intricacies of various AI techniques, including unsupervised, semi-supervised, supervised, and reinforcement learning, as well as Large Language Models (LLMs) and Foundation Models (FMs).
The Industrial AI Report also dispels myths and misconceptions. One common myth is that AI implementation requires substantial upfront investment. While initial costs can be high, the long-term benefits often outweigh these costs. Another prevalent misconception is that AI will eliminate jobs. However, while AI may automate certain tasks, it also creates new roles and opportunities.
It discusses the shift in priorities from Industrial Metaverse to Industrial AI, emphasizing the potential of AI to drive efficiency and innovation in industries. For more on this particular topic read my blog on how Industrial AI is paving the way for Industrial Metaverse(s).
Industrial organizations can leverage ARC's Industrial AI Impact Assessment Model used by ARC's own team of Analysts, to guide their own AI evaluation and implementation process. This model offers a structured approach to assess the potential impact of AI on various aspects of the organization, including operations, strategy, and workforce.
As we continue to explore the potential of generative AI and other breakthroughs in industrial AI, collaboration and knowledge sharing become increasingly important. We invite you to join us in this journey, sharing your questions, experiences, learnings, and solutions.
The future of Industrial AI is promising, with its potential to transform industries and societal structures. By deepening our understanding and effectively applying AI technologies, we can unlock their true potential in the industrial realm.
For more information or to contribute to Industrial AI research, please contact Colin Masson at cmasson@arcweb.com.