
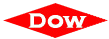




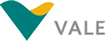

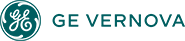




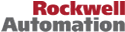

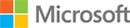
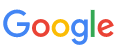

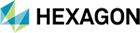


A variety of factors are influencing the growing awareness and interest in analytics in EAM by maintenance groups. Today’s industrial organizations see the value that effective data management, KPIs, and both predictive and prescriptive analytics can bring. “After-the-fact” reports based on historic in-formation alone are no longer adequate. To compete effectively in today’s hyper-competitive industrial environments, organizations need to provide users with actionable, real-time (or near-real-time) information.
This is particularly true for the maintenance groups tasked with keeping a company’s manufacturing assets available and performant. As a result, analytics are becoming critical for effective enterprise asset management.
Today’s maintenance organizations have access to much foundational data. These include asset data, labor data, and job plan information, preventive and corrective maintenance scheduling, and sensor-based condition monitoring data. All these data, whether structured from relational data-bases or data warehouses; or unstructured, text-based information found in work requests, work orders, e-mail messages, or other forms must be mined, evaluated, and analyzed.
While, in the past, analytics were the sole domain of corporate data scientists, many of today’s newer analytics solutions were designed for use by plant-level maintenance and operations staffs. This has helped “democratize” analytics to a large degree, making these solutions much more accessible.
Analytics come in a wide variety of categories and variations. They can range from views of historic data to data mining and analyses of connected, real-time, and near-real-time information. Examples of common analytics categories include:
For many maintenance organizations, existing KPIs are a good foundation for areas that may need deeper analysis. Combined with analytics, maintenance KPIs can provide even greater visibility into asset condition and health.
Examples can include line and machine uptime, equipment availability, maintenance costs vs. budgets, labor costs and hours vs. budget, preventive maintenance performance summaries, overall maintenance quality, and the percentage of unplanned and emergency work. Other KPIs can include such measures as mean-time-to-failure (MTTF), mean-time-to-repair (MTTR), equipment downtime statistics, and overall equipment effective-ness (OEE).
ARC Advisory Group clients can view the complete report at ARC Client Portal
If you would like to buy this report or obtain information about how to become a client, please Contact Us
Keywords: Descriptive Analytics, Predictive Analytics, Key Performance Indicators (KPIs), Business Intelligence (BI), Enterprise Asset Management (EAM), ARC Advisory Group.